10 Artificial Intelligence terms everyone should know
11-07-2024
Unravel the most advanced concepts of Artificial Intelligence with this essential list of terms.
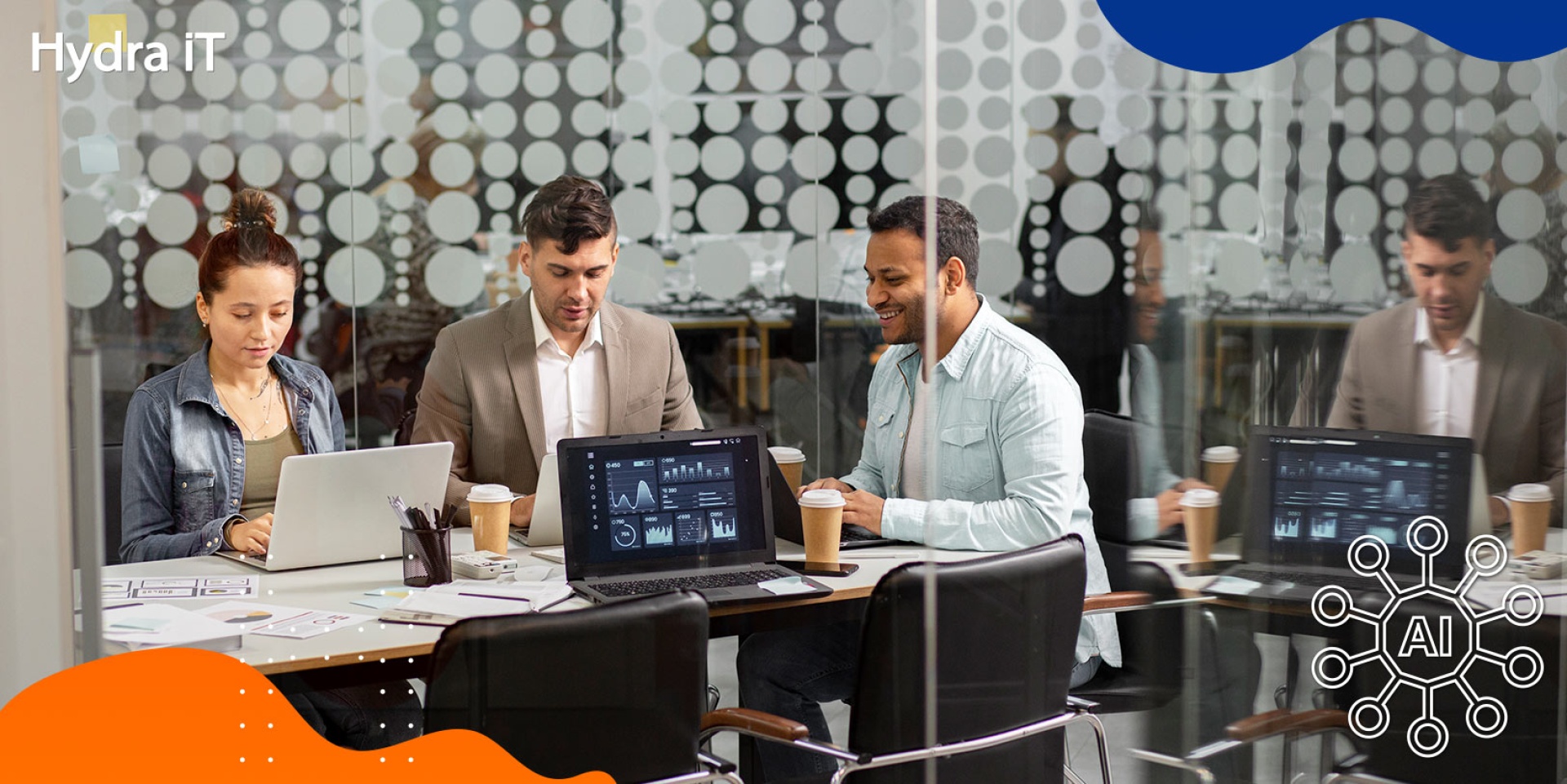
Since generative Artificial Intelligence (AI) became popular in late 2022, most people have understood this technology and how it uses natural language to help interact more easily with computers. However, as AI continues to evolve, its lexicon is also expanding. Do you know the difference between large and small language models? Do you want to understand all the terms so you can understand conversations with your friends?
Microsoft has put together a set of 10 advanced AI terms to keep you up to date with all the latest technology and concepts.
- Reasoning/Planning
- Training/inference
- SLMs/Small Language Models
Small language models, or SLMs, are "pocket versions" of large language models, or LLMs. Both use machine learning techniques to recognize patterns and relationships in language, producing realistic and natural responses. However, while LLMs are larger and require a lot of computing power and memory, SLMs, such as Phi-3, are trained on smaller, selected data sets with fewer parameters. They are therefore more compact and can even be used offline, without an internet connection. This makes them more compact and capable of operating offline, making them ideal for applications on devices such as laptops or cell phones.
- Rationale
Generative AI systems can compose stories, poems, and jokes, as well as answer research questions. However, sometimes they have trouble separating fact from fiction or have outdated data, resulting in inaccurate answers, called hallucinations. Programmers work to improve the accuracy of AI through grounding, linking, and anchoring the model to tangible data and examples to produce more contextualized, relevant, and personalized results.
- Augmented Retrieval Generation (RAG)
When programmers give an AI system access to a grounding source to help it be more accurate and current, they use a method called Retrieval Augmented Generation or RAG. The RAG standard saves time and resources by adding extra knowledge without the need to reprogram the AI system.
- Orchestration
The orchestration layer in AI programs directs its tasks in the correct order to obtain the best response. This layer can also use the RAG standard, searching for new information on the Internet to improve responses. It works like a conductor who coordinates different instruments to produce the music as the composer planned.
- Memory
Current AI models have no memory but they can follow instructions that help them "remember" information in each transaction, such as temporarily storing previous questions and answers in a chat and including that context in current requests. Developers are experimenting with levels of orchestration to help AI decide whether it needs to temporarily remember steps (short-term memory, like a sticky note) or whether it would be useful to store information for longer in a more permanent location.
- Transformation models and diffusion models
For decades, AI systems have been taught to understand and generate language, but a crucial recent advance has been the transformative model. Among generative AI models, transformers stand out for their ability to quickly understand context and nuances, being able to continuously predict the next step and generate eloquent text. On the other hand, diffusion models, known for creating images, use a gradual and meticulous process to distribute pixels from random positions until they reach the desired image, continuously adjusting until they achieve the desired result.
- Frontier models
Frontier models are large-scale systems that push the boundaries of AI, performing various tasks with advanced and surprising capabilities. Technology companies such as Microsoft have created a Frontier Model Forum to share knowledge.
- GPU
A GPU, or Graphics Processing Unit, is essentially a boosted calculator, originally developed to render complex graphics in video games and now used as a powerful computing engine. With multiple cores that perform calculations in parallel, GPUs are crucial for AI applications, both in training and in performing inferences. Advanced AI models are often trained on huge clusters of interconnected GPUs, such as those used in Microsoft's Azure data centers, which represent some of the most powerful computers ever built.
Stay tuned to the Hydra iT blog for all the latest technology news!
Source: Microsoft News
Stay tuned to the Hydra iT blog for all the latest technology news!
Source: Microsoft News
Share